Program Highlights
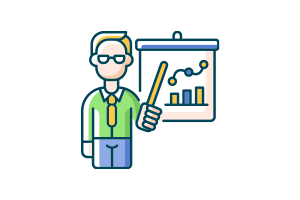
Top-Notch Faculty
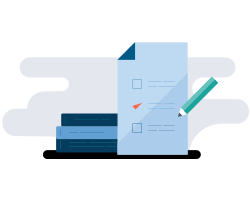
Exhaustive Course Curriculum
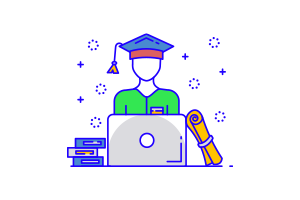
Job Readiness
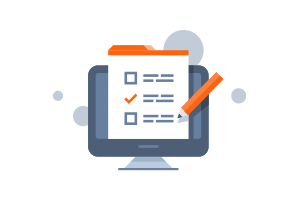
Real-life Projects
Skills Covered
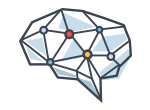
Deep Learning
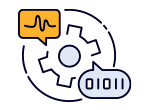
Natural Language Processing
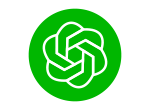
ChatGPT
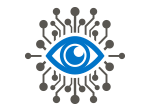
Computer Vision
Tools and Technologies
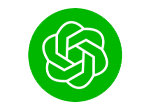
ChatGPT
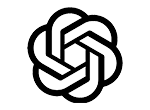
DALL-E 2
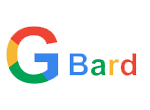
Gbard
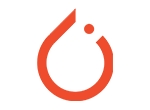
Pytorch
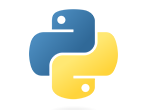
Python
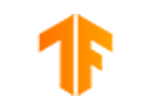
TensorFlow
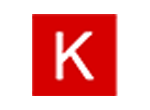
Keras
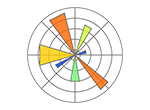
Matplotlib
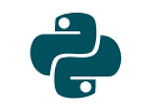
NLTK
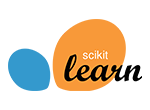
Scikit-learn
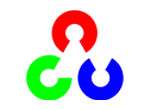
OpenCV
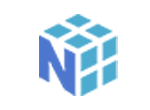
NumPy
Course Overview:
This Artificial Intelligence (AI) course is a 130-hour training program meticulously designed to help working professionals and freshers know the framework, intertwined concepts, utility tools, and other best practices in Artificial Intelligence, inside out! The elaborate curriculum and other highlights outlined in this brochure explain in detail why you should immediately embark on your learning journey with us. The modules cover a vast array of Artificial Intelligence tools like ChatGPT, DALL.E 2, Gbard, Pytorch, Python, Tensor Flow, Keras, OpenCV, NumPy, etc. With instructor-led theory classes integrated with labs and capstone projects, you’re sure to master Artificial Intelligence (AI)!
Advanced Certification Program in Data Science and AI for Digital Transformation from IITM Pravartak:
ExcelR, in association with IIT Madras, brings to you an add-on certification for your Artificial Intelligence Course.
This certification program provides you with:
- 15+ Hours of Interactive Live-Virtual Sessions by professors of IIT Madras.
- Optional 2-day Campus Immersion in the beautiful, state-of-the-art IIT Madras.
- A prestigious IIT Madras Pravartak Certificate.
What is the certification process?
During the period of your course, interactive live-virtual sessions will be conducted by professors of IIT Madras. An optional campus immersion will also be planned, whereby a slot will be created, and you will travel to Chennai for a two-day experience at the IIT Madras campus. Post training, you will take a short quiz on the topics discussed in the session, which will unlock your Advanced Certification in Data Science and AI for Digital Transformation from IITM Pravartak.
Projects
-
- Beginner Level
- AI project to develop a model to classify text data, such as social media posts or customer reviews, into sentiments like positive, negative, or neutral. The project involves collecting and pre-processing text data, using tools like NLTK or spaCy for tasks like tokenization and lemmatization. Feature extraction is achieved through techniques such as TF-IDF or word embeddings (e.g., Word2Vec). Machine learning or deep learning models, implemented using TensorFlow, PyTorch, or Scikit-learn, are trained, and tested on this data. The project offers practical experience in natural language processing and sentiment classification.
- Beginner Level
- A beginner level GAN (Generative Adversarial Network) project, for the fashion industry, involves creating a model that can generate new, realistic clothing designs. In this project, the GAN consists of two parts a Generator, which produces images of clothing items, and a Discriminator, which evaluates whether these images are real or generated. The training process involves feeding the network with a dataset of various clothing images, allowing the Generator to learn and create novel designs while the Discriminator improves its ability to distinguish between real and synthetic images.
- Intermediate Level
- One of the earliest goals for computers was the automatic translation of text from one language to another. Automatic or machine translation is perhaps one of the most challenging artificial intelligence tasks given the fluidity of human language. Objective is to translate phrases in one language to another language. Any dataset from the web can be used for this project. We get to work using transformer self-attention models for example T5 model (text to text transformer model) from Hugging face library. We also learn how to design encoder and decoder LSTM models for translation.
- Intermediate Level
- Explore and identify at least 10 CNN architectures/ models which are used in image classification and object detection problems. Work on understanding these architectures by reading literature, papers etc and do comparative analysis of these models on image classification and object detection datasets. We learn how to design and train a deep learning CNN model for image classification and object detection problems. We also learn how different CNN deep learning models are and find out which is the best model.
- Advanced
- The objective is to caption the image in your dataset (Download it from web) and come up with a suitable title for the image and then try it on a video and see if it can work on video as well. You are free to use a mix of CNN and RNN, LSTM models for this project. We learn how to prepare photo and text data for training a deep learning model, how to design and train a deep learning caption generation model and how to evaluate a train caption generation model and use it to caption entirely new images and photographs.
- Advanced
- Data extraction from YouTube using API's. Work on clear exploratory data analysis. Build recommendation engine using that video contents. Need to deploy the entire setup. Create an application for your model. Learning outcome, we will know how to extract data using YouTube using API's and how to design and train a deep learning video recommendation model and how to evaluate a deep learning video recommendation model and deployment.
Case Studies
- A CNN (Convolutional Neural Network) project focused on e-commerce product image classification involves designing a neural network to automatically categorize product images into predefined classes. This system uses CNN's ability to extract features and recognize patterns in images, making it highly effective for visual data analysis. The classification process helps in organizing and managing large e-commerce inventories, improving search and recommendation systems.
- Another friendly GAN project involves generating human faces using the CelebA dataset, a collection of celebrity face images. The goal is for the Generator to create new, realistic-looking faces, and for the Discriminator to differentiate between these generated faces and real images from the dataset.
- A chatbot project using neural networks involves developing an intelligent conversational agent capable of understanding and responding to user queries in natural language. The neural network, typically a form of deep learning model like LSTM (Long Short-Term Memory) or Transformer, is trained on large datasets of conversational text to learn language patterns and context. This chatbot can be integrated into various platforms, such as customer service portals, to provide automated, 24/7 assistance.
- Difficulty Level
- Build a Reinforcement learning model to solve a straightforward problem like navigating a maze. In such a project, the Q-learning algorithm, a form of model-free reinforcement learning, is used to teach an agent how to make optimal decisions to maximize a cumulative reward in a defined environment. The agent learns by exploring the environment, receiving feedback in the form of rewards or penalties, and updating a Q-table that maps state-action pairs to rewards.
Career Progression and Salary Trends
Learning Path
Why ExcelR
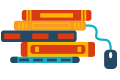
Industry-Based Course Curriculum
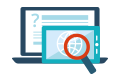
Value Added Courses: Python,ChatGPT,Prompt Engineering,Generative AI and MLOps
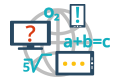
Hands-on with
20+ Assignments
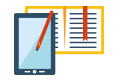
Job Readiness Program with our 2000+ partner companies
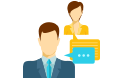
Support through WhatsApp, Calls, & Emails
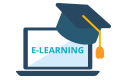
Lifetime eLearning Access
Course Curriculum
- Basic Concept
- ML and AI introduction
- Applications of ML and AI
- Python
- Basic Programming
- NLP Libraries - Spacy & Gensim
- OpenCV & Tensorflow, Keras
- Basic Statistics
- Sampling & Sampling Statistics
- Inferential Stats : Hypothesis Testing
- Calculus
- Derivatives
- Optimization
- Linear Algebra
- Function
- Scalar-Vector-Matrix
- Vector Operation
- Probability
- Space
- Probability
- Distribution
- Unsupervised
- Unsupervised K-Means & Hierarichal Clustering
- Linear Regression
- Logistic Regression
- Evaluation Metrics
- Train,Test & Validation Distribution
- Supervised
- Gradient Descent
- Decision Tree & KNN
- Random Forest | Bagging & Boosting
- Introduction
- Intro
- Deep Learning Importance [Strength & Limiltation]
- SP | MLP
- Feed Forward & Backward Propagation
- Neural Network Overview
- Neural Network Representation
- Activation Function
- Loss Function
- Importance of Non-linear Activation Function
- Gradient Descent for Neural Network
- Practical Aspect
- Train, Test & Validation Set
- Vanishing & Exploding Gradient
- Dropout
- Regularization
- Optimization
- Bias Correction
- RMS Prop
- Adam,Ada,AdaBoost
- Learning Rate
- Tuning
- Softmax
- Image preprocessing
- Introduction to Computer Vision ,Image, image transformation, filters, noise removal, edge detetction, non-max suppression , hysterisis
- Advanced CNN concepts -1
- "Object detection concepts, Bounding box, object detection models, landmark detection, RCNN, fast RCNN, faster RCNN, mask RCNN, YOLO pre-trained models, transfer learning , segmentation concepts"
- Advanced CNN concepts -2
- Advanced CNN models applications, face detection and recognition, different techniques in face recognition, style transfer
- Speech Processing
- "Introduction, Automated Speech Recognition (ASR) "
- Speech Synthesis
- text to speech conversion, voice assistant devices, building alexa slkills
- Autoencoders & Decoders
- Basics of autoencoders, different types of autoencoders, applications with examples , variational autoencoders, intro to Gen AI
- Generative Adverserial Networks (GAN's)
- GAN basics and foundations, upsampling , GAN models, evaluate GAN Models, inception score, frechet inception distance, GAN loss functions
- GAN's different types
- Conditional GAN, Info GAN, Auxillary GAN etc, applications
- GAN use cases
- Image translation applications, cycle GAN concepts and implemenations
- Reinforcement Learning
- Intro to RL, Q learning, Exploration , exploitation
- Reinforcement learning applications
- Work with deep RL libraries, openai gym library, policy gradient concepts, Actor-critic methods, Proximal policy Optimization (PPO) and related concepts
- Forecasting deep learning
- ARIMA, Deep learning models for forecasting (RNN, LSTM , Transformer applications)
- Basic NLP concepts & models
- "Introduction to Text Mining,VSM, word embeddings applications, RNN , GRU, LSTM models, Intro to Transformers, Attention (Elmo, BERT , T5)"
- "Text Mining & NLP applications, Web Scraping"
- "Word clouds and Doucument Similarity using cosine similarity, Named Entity Recognition, machine translation using hugging face libraries, Emotion Mining using different libraries, web scraping"
- Naive Bayes
- "Text classification using Naïve Bayes, frequqentists vs bayesian , apriori, posteriori distributions Bayesian estimators: posterior mean, posterior median"
- "Advanced NLP models , Generative AI using LLM's"
- "Intro to Transformers & Attention (Single Head,Multi Head) , pretrained models (GPT, BERT ,BART, T5) models with applications , examples using python Intro to Different types of Transformer encoder models- Basic BERT, RoBERTa, DistilBERT etc. Intro to Different types of Transformer decoder models-GPT, GPT2, other variants of GPT etc, GPT progress, calling OPENAI api's , LLM playgrounds Intro to Different types of Transformer sequence to sequence models-BART, T5"
Value Add Courses
- Introduction
- Python Introduction - Programing Cycle of Python
- Python IDE and Jupyter notebook
- Variables
- Variables
- Data type
- Code Practice Platform
- create , insert , update and delete operation , Handling erros
- Operators
- Operator -Arthmatic ,comparison , Assignment ,Logical , Bitwise opeartor
- Decision making - Loops
- Loops
- While loop, for loop and nested loop
- Number type conversion - int(), long(). Float ()
- Mathametical functions , Random function , Trigonometric function
- Sting
- Strings- Escape char, String special Operator , String formatting Operator
- Build in string methods - center(), count()decode(), encode()
- List
- Python List - Accessing values in list, Delete list elements , Indexing slicing & Matrices
- Built in Function - cmp(), len(), min(), max(), list comprehension
- Tuples
- Tuples - Accessing values in Tuples, Delete Tuples
Contact Our Team of Experts